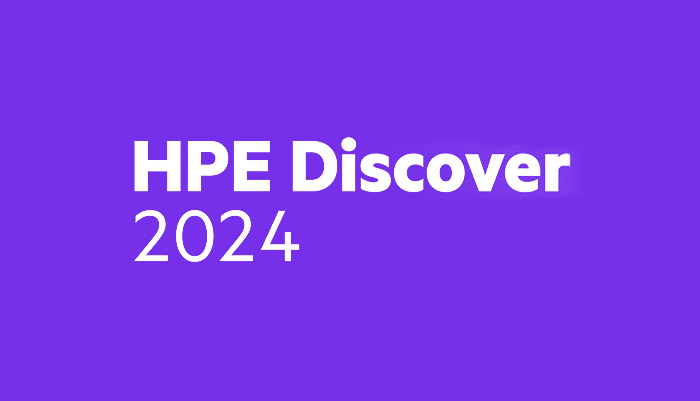
Reshaping storage architecture in the age of AI: HPE Discover 2024
Published on •3 mins Last updatedAs an HPE Technology Partner, we were thrilled to be a Startup Innovation Sponsor at this year's HPE Discover 2024 Las Vegas event, speaking to attendees about how to build more resilient storage solutions while embracing future innovations.
Top of the agenda this year was how storage infrastructure needs to evolve to capitalize on AI. And the importance of load balancers in underpinning this shift. Here's how..
An end-to-end storage solution for the future
Together, HPE and Loadbalancer provide a one-stop-shop for customers requiring end-to-end storage solutions, delivering a seamless end-user experience, guaranteed uptime, and consistent data access.
As Kai Wai Leung, HPE's GreenLake Flex Cloud ISV Partner explained:
“Having validated with most storage applications is a key advantage of partnering with Loadbalancer.org. And, through our GreenLake Flex program, customers now have access to a full spectrum of network solutions including high availability.”
Because storage environments are becoming more and more complex, partnering not only reduces the complexity for customers, but also reduces operational costs.
Loadbalancer's work with partners such as HPE, Scality, Cloudian, and CTERA over the last twenty years, as well as resellers and end-users, means we're able to provide specialist insights on the unique requirements of load balancing individual storage ecosystems and AI workflows.
As such, we were invited to share these insights at HPE Discover 2024.
How load balancers facilitate the storage and protection of AI data
It’s not just storage applications themselves that need load balancing; it’s also the surrounding ecosystem. Storage points into a number of different architectures and key client-side applications, so it's important these end-to-end workflows are also load balanced in order to retain smooth data access, storage, and analysis. And never was this more true than for artificial intelligence (AI).
The sheer volume of data that forms the life-blood of AI, demands more nuanced load-balanced storage solutions deployments to future-proof data demands, provide more resilient workflows, and ensure fast access to the required data.
For more on the technical reasons why the AI workloads themselves rely on high-performance object stores, check out this great MinIO article: The Real Reasons Why AI is Built on Object Storage.
So. Having established the importance of object storage for AI, what role do load balancers play in optimizing these workflows?
Here are just three examples:
1. Scalable AI storage infrastructure
AI requires large data sets to be processed in a short timeframe, which drives sizeable storage demands. The management of these data sets requires solutions such as object storage that can scale limitlessly within a single namespace, with a modular design allowing capacity to be added at the moment it's needed — not ahead of time.
But how do these object storage solutions themselves scale?
Load balancing reduces the burden of scale-out with simple autoscaling of a storage cluster or the easy addition of a new node to the storage cluster.
2. End-to-end resilience to protect your AI workloads
Backing up a multi-petabyte AI data set is not always feasible. In other words, it would likely take too long or be cost-prohibitive. But you can't leave it unprotected and risk falling foul of stringent Service Level Agreements, or potential data loss in the event of downtime.
How do object storage solutions overcome this problem?
Object storage environments require load balancers to provide redundancy, meaning storage systems can tolerate multiple node failures, or even the loss of an entire data center.
3. Fast access to complex, hybrid AI storage architecture
AI systems often rely on a complex web of hybrid architecture, from data centers to the cloud's edge, and AI storage ecosystems must include the right mix of technologies to facilitate seamless access to AI data.
While some aspects of the AI workflow will likely reside in the cloud, much of it will remain in the data center because it can be accessed faster, it's cheaper to analyze, or because compliance regulations require it. This therefore requires the seamless flow of data from local data centers to the cloud, and back again.
With load balancing, it's possible to ensure even traffic distribution, reduced response times, and health monitoring, meaning more efficient resource utilization and superior performance across hybrid AI workflows.
Conclusion
AI requires specialized or enhanced storage solutions, designed specifically to provide high-performance, scalable, and resilient infrastructure.
We ensure that AI environments are highly available, scalable, and have sufficient failover capabilities to keep your data flowing, even during data center failures.